Analyze Sentiment
Use our free sentiment analysis tool to discover the emotional tone of your text. This NLP-powered tool categorizes text.
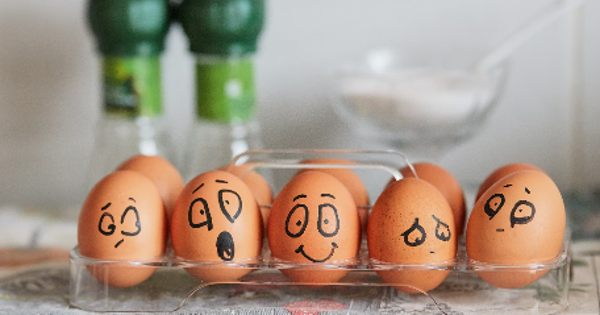
Evaluate Content Sentiment
Enter the URL below and analyze the sentiment of your webpage content to gain insights and enhance your messaging.
What is sentiment analysis?
How can I use sentiment analysis to improve SEO?
Discover how you can use sentiment analysis to optimize your online presence
- Content optimization: By analyzing sentiment in user-generated content, such as reviews, comments, and social media posts, you can identify keywords and topics that resonate positively with your audience. This insight can inform your content creation strategy, helping you produce relevant and engaging content that attracts more traffic and improves search engine rankings.
- Reputation management: Monitoring sentiment around your brand allows you to identify and address negative sentiment promptly. By responding to negative feedback and addressing customer concerns, you can mitigate the impact on your brand reputation and improve trust and credibility, which are important factors for SEO.
- User experience optimization: Sentiment analysis can help you understand how users perceive your website or digital assets. By analyzing sentiment in user feedback and behavior, you can identify areas for improvement in user experience, such as navigation, content quality, and overall satisfaction. Optimizing the user experience can lead to higher engagement, longer time spent on site, and lower bounce rates, all of which are positive signals for search engine rankings.
- Keyword research: Sentiment analysis can uncover new keywords and phrases that reflect user sentiment and preferences. By incorporating these keywords into your SEO strategy, you can attract more targeted traffic and improve the relevance of your content to user intent.
- Competitor analysis: Analyzing sentiment around your competitors' brands and content can provide valuable insights into market trends, customer preferences, and areas of opportunity. By staying informed about competitor sentiment, you can identify gaps in the market and tailor your SEO strategy to capitalize on them.
How to incorporate sentiment analysis in my content?
Incorporating sentiment analysis into your content strategy can improve your SEO efforts by making sure that your content resonates with your audience.
Here are some guidelines:
- Understand your audience: Use sentiment analysis to gain insights into your target audience's preferences, emotions, and sentiment towards different topics, products, or services. This understanding will help you adapt your content to meet their needs and expectations.
- Use sentiment-driven keywords: Identify keywords and phrases that reflect positive sentiment and incorporate them into your content, including headings, titles, meta descriptions, and body text. This will help your content rank higher in search engine results pages (SERPs) and attract more traffic from users with positive intent.
- Address user concerns: Monitor sentiment analysis to identify any negative sentiment or concerns expressed by your audience. Use this feedback to address any issues or gaps in your content, products, or services and provide solutions that meet their needs and expectations.
- Create engaging content: Analyze sentiment to understand which types of content resonate most with your audience and generate positive engagement. Use this insight to create more engaging and compelling content, such as videos, infographics, or interactive elements, that encourages users to interact and share.
- Monitor sentiment trends: Continuously monitor sentiment analysis to track changes in user sentiment and identify emerging trends or topics of interest. Use this information to adjust your content strategy accordingly and stay ahead of the curve in your industry or niche.
- Measure performance: Use sentiment analysis tools to measure the impact of your content on audience sentiment and engagement. Monitor key metrics such as sentiment score, engagement rate, and conversion rate to gauge the effectiveness of your content and make data-driven decisions for future optimization.
Common mistakes in using sentiment analysis
When using sentiment analysis, it's important to be mindful of common mistakes to ensure accurate and meaningful results.
Here are some common mistakes to avoid:
- Ignoring context: Sentiment analysis algorithms may struggle to accurately interpret context, leading to misinterpretations of sentiment. It's essential to consider the context in which language is used to avoid misclassification of sentiment.
- Over-reliance on automated tools: While sentiment analysis tools can provide valuable insights, they are not infallible and may produce inaccurate results, especially when dealing with complex or nuanced language. It's essential to complement automated analysis with human judgment and interpretation.
- Neglecting customization: Generic sentiment analysis models may not capture the nuances of specific industries, audiences, or languages. Customizing sentiment analysis models to your specific needs and domain can improve accuracy and relevance.
- Failing to account for sarcasm or irony: Sentiment analysis algorithms may struggle to detect sarcasm, irony, or other forms of figurative language, leading to inaccurate sentiment analysis results. It's important to consider the possibility of such language and interpret results accordingly.
- Disregarding data preprocessing: Preprocessing textual data, such as removing stopwords, punctuation, and special characters, is crucial for improving the accuracy of sentiment analysis results. Failing to preprocess data adequately can lead to noise and errors in sentiment analysis.
- Neglecting human validation: While automated sentiment analysis can provide valuable insights at scale, human validation is essential for verifying the accuracy of results and identifying any misclassifications or errors. It's important to incorporate human judgment and validation into the sentiment analysis process.
We use essential cookies to make our site work. With your consent, we may also use non-essential cookies to improve user experience and analyze website traffic. By clicking "ACCEPT", you agree to our website's cookie use as described in our Cookie Policy.